Artificial intelligence (AI) is making waves in the world of drug discovery. Rajiv Anand, founder and CEO of the firm Quartic.ai, explains how the tool could also make pharmaceutical manufacturing more efficient.
The use of AI in drug discovery is attracting big investor interest; around $1.4 billion so far this year has gone to startups focused on drug discovery. One of the reasons for this excitement is that AI has the potential to speed up the process from years to months.
However, AI has other potential in the biotech industry aside from drug discovery. For example, AI could be applied in the manufacturing process of new medicines.
To succeed in the market, a drug must be manufactured at a commercial scale. The many complex steps needed to scale up the pharmaceutical manufacturing process — such as obtaining equipment, analysing manufacturing data and screening for variability in the manufacturing yield — are known as process development (PD).
In addition, firms must invest in manufacturing R&D to meet the U.S. Food and Drug Administration (FDA)’s safety and quality guidelines, known as good practice (GxP) compliance.
In an interview, Rajiv Anand, founder and CEO of the pharmaceutical manufacturing AI specialist Quartic.ai, explained how AI and machine learning (ML) are becoming increasingly popular as biotech companies strive to speed up PD and get their new medicine to market.
Why do current pharmaceutical manufacturing strategies need improving, and how can AI make it easier?
Existing workflows and tools, many of which are still manual or paper-based, aren’t able to support the pace of pharma product development, the number of products under development at any time, and increased use of biologics as well as cell and gene and other novel therapies.
On the development side, PD capacity is becoming a bottleneck and PD practitioners are under severe pressure. Within manufacturing tech transfer, scale-ups and general capacity creation and optimization are also highly inefficient. Deviation investigations and improvements still take several weeks and sometimes even months.
AI can make process and operational qualifications more efficient – sometimes reducing the number of engineering and qualification runs by more than half. Variability investigations can be automated with AI, and the advanced technology can be used for autonomous control with predictable titers and quality.
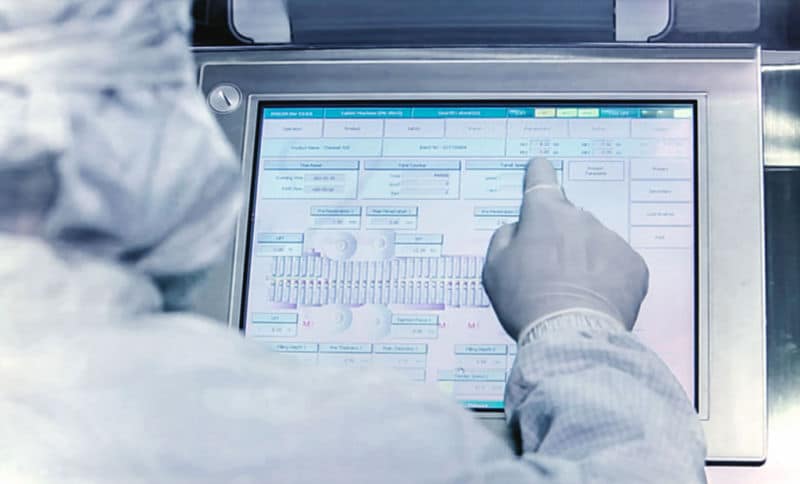
What are the biggest challenges of implementing AI in pharmaceutical manufacturing, and how is Quartic.ai working to solve them?
The first is the availability of ready-to-use, contextual data both for posterior analysis, real-time analysis, and predictive operations with AI. Even those pharma companies that have invested a lot in data science and analytics teams struggle with this.
These teams report spending more than 3–4 times more time in data preparation than in building models. Sometimes, AI models are built to work on streaming (real-time) data, but are tested using static historical data, giving the user a false promise of high accuracy, but poor performance when deployed on streaming data.
Quartic.ai provides a complete contextual data pipeline for use in streaming and batch AI models. This completely eliminates the data access and preparation tasks and allows the analytics teams to focus on model building. The built models can be tested on streaming data to ensure they will perform as tested.
The second is the availability of large datasets. Most machine learning and AI algorithms have their origins in big data. Pharma manufacturing generally lacks large amounts of valuable data, and this problem is even more pronounced in PD. Quartic.ai has developed and deployed small-data/sample-efficient AI to specifically address this challenge.
The third is the model deployment pipeline and integration. Once good AI models have been built, they need to be deployed on a reliable pipeline that can be monitored for performance, versioning etc. as well as integrated with the process control and quality systems. Quartic.ai provides an ML pipeline that is tightly integrated with the incoming data, supports models built using Quartic.ai’s ML frameworks, and integrates the output of the models to visualization systems, process control systems and quality management systems.
Both the data pipeline, as well as the model pipeline are GxP-compliant and provided with validation protocols.
How are investor attitudes changing regarding the use of AI in manufacturing?
The growing need and breadth of pharmaceutical products is of great interest to investors. There have been some significant investments in AI technology companies supporting discovery.
In the last 2–3 years, particularly with the attention that supply chain disruption has received, investor interest in companies that can accelerate the manufacture and delivery of these products and increase manufacturers’ profitability has been building up fast.
With the lack of returns from consumer, social and Web3 and crypto technology companies, investors are looking at the intersection of AI, manufacturing and pharma as a major growth area where they can deploy stranded capital.
Can you explain how the use of AI in pharmaceutical manufacturing might impact regulatory processes? How well are regulatory agencies keeping up with the technology?
Often, people assume that AI cannot be used in a regulated environment, or it is hard to validate because it is a “black box.” The reality is that validating an application or a process using AI is no different than the validation processes used for traditional methods like statistical process control (SPC).
Of course, the regulators want the manufacturer to use the proper validation protocols required in GxP applications. The problem is that when these applications are built using ad hoc, “one-off” models and workbenches, validation is difficult.
When you take a product- and platform-centric approach, it is a lot easier. Well-documented validation protocols that support each step allow the user to easily deploy them in a regulated process.
As early as 2015, with the formation of the Emerging Technologies Program (ETP) of the FDA, regulators have encouraged the use of advanced technologies like AI. During the pandemic, when we faced substance shortages, the regulators were even more supportive, provided the proper validation protocols were followed.
Of course, acceptance is a lot faster when AI is applied to a novel method of manufacture or process.